Artificial Intelligence for Medicine: Can the Seemingly Impersonal Become Quite Personal Indeed?
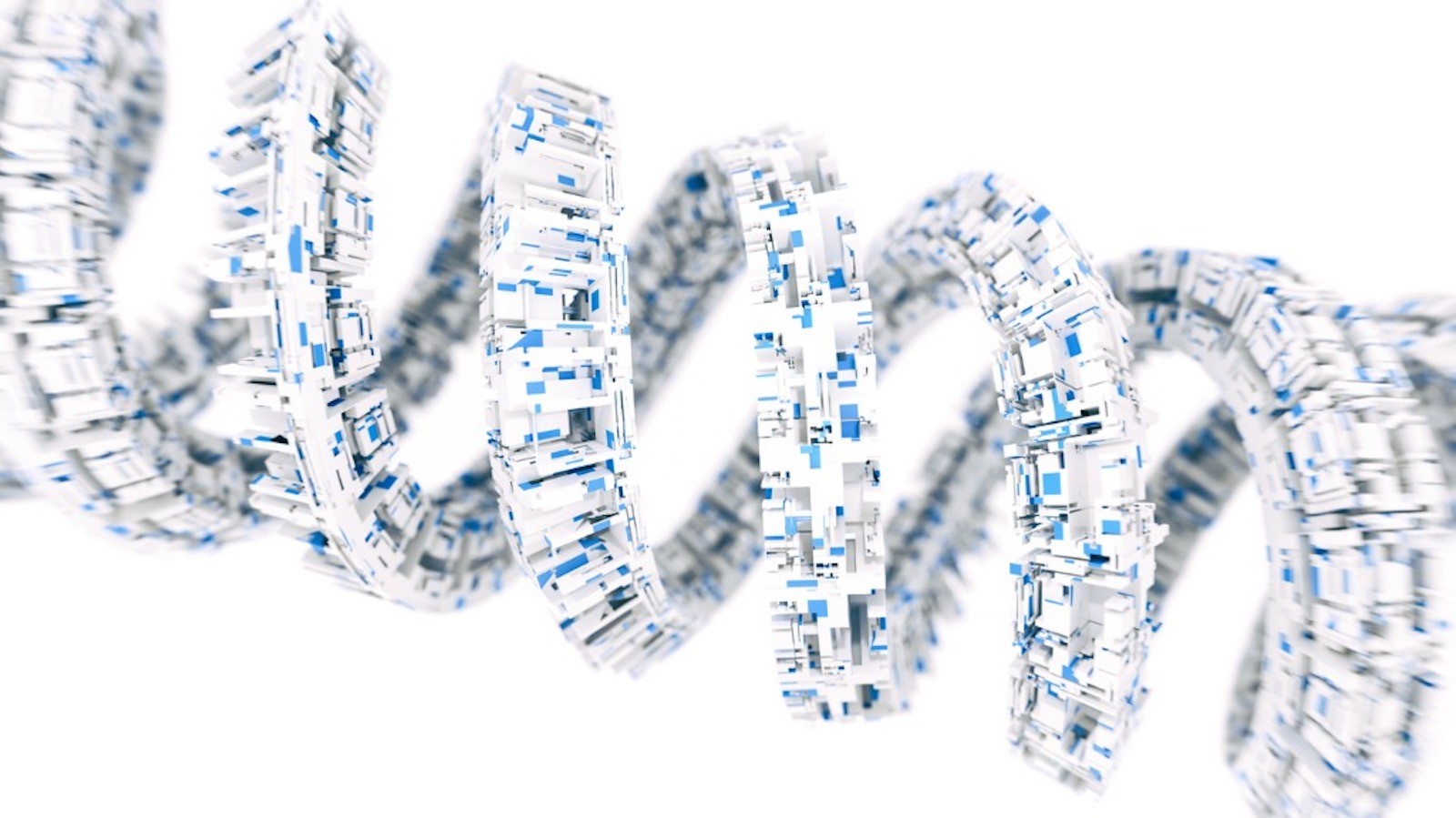
In a recently published article in the Annals of Thoracic Surgery, clinical investigators from The Texas Heart Institute and Baylor College of Medicine hypothesized that artificial intelligence (AI) and “machine learning” could be used to predict the risks and costs associated with various cardiac surgery procedures. In collaboration with Houston digital health technology company InformAI, the team developed, trained, and tested a machine-learning computer algorithm that accurately predicted three surgery-related outcomes: death, major morbidity (i.e., health problems arising shortly after surgery), and high hospitalization costs.
As computers become more and more powerful and massive “big data” datasets are collected, AI and machine learning have come to the fore. Artificial intelligence is a computer system’s ability to think like a human and problem-solve on its own. Machine learning is how that computer system develops its intelligence by using available data to train itself.
While experienced physicians rely on years of training and practice to understand and treat their patients, AI and machine learning can detect unknown and unobvious interactions among millions of data points. Such enhanced knowledge can inform clinician understanding about patient status, optimal treatments, inherent risk, and probable outcomes, including surgery outcomes.1,2 Even better, with higher computational capabilities, machine learning models can be updated constantly as more data are collected, enabling ever-quicker individualized and institution-specific predictions.
The algorithm developed for this study investigated 83 preoperative input parameters (i.e., patient, surgeon, and institutional factors that could affect outcomes) and operative outcomes from almost 5,000 patients in the Society of Thoracic Surgeons (STS) Adult Cardiac Surgery Database at Baylor College of Medicine; 56% of the patients had undergone coronary artery bypass surgery, 42% had had valve surgery, and 19% had had aortic surgery. Major morbidity was defined as having at least one of the following: reoperation for any cardiac reason (6% of patients), kidney failure (4%), deep sternal wound infection (1%), prolonged ventilation or intubation (6%), or stroke (3%).
Data from 80% of the patients were used to train the algorithm, and data from the remaining 20% were used to test the final model. After running the algorithm 25,000 times in the training phase, only 25 of the initial 83 input parameters were found to be necessary for preoperatively predicting mortality (95% accuracy), major morbidity (71% accuracy), and high hospitalization cost (84%). Preoperative hematocrit, platelet, hemoglobin A1c, and creatinine levels, white blood cell count, age, weight, height, liver dysfunction, and needing an aortic procedure consistently predicted these adverse outcomes, suggesting that closer attention should be paid to preoperative laboratory results. Importantly, the algorithm performed as well or better than the STS risk models available for some—but not all—cardiac surgery procedures,3 and it uses only about a third of the input parameters that STS risk calculators typically require.
Machine learning models enable risk prediction based on local data and can continuously incorporate new data in real time. They may also be better than the human brain at incorporating nonlinear data and complex interactions (e.g., surgeon-specific characteristics, operative volume) into the prediction model, which means that machine learning–based analysis of electronic health records may identify factors not previously considered in analyses of surgical registry data. This makes the machine learning algorithm an attractive option for accurate, personalized risk prediction. Using AI and machine learning to interrogate large repositories of clinically relevant electronic data could significantly improve patient care, cost effectiveness, and reimbursement.4
The human touch will always be needed in patient-doctor interactions. Machine learning and AI are simply additional tools available to physicians seeking to improve diagnosis and treatment and to clarify probable risks for a wide range of cardiac surgical procedures.
References
- Johnson KW, Torres Soto J, Glicksberg BS, et al: Artificial intelligence in cardiology. J Am Coll Cardiol 71: 2668-2679, 2018.
- Kilic A: Artificial intelligence and machine learning in cardiovascular health care. Ann Thorac Surg 109: 1323-1329, 2020.
- Kilic A, Goyal A, Miller JK, et al: Predictive utility of a machine learning algorithm in estimating mortality risk in cardiac surgery. Ann Thorac Surg 109: 1811-1819, 2020.
- Bertsimas D, Dunn J, Velmahos GC, Kaafarani HMA: Surgical risk is not linear: derivation and validation of a novel, user-friendly, and machine-learning-based Predictive OpTimal Trees in Emergency Surgery Risk (POTTER) calculator. Ann Surg 268: 574-583, 2018.
Read Report
Zea-Vera R, Ryan CT, Navarro SM, Havelka J, Wall MJ Jr, Coselli JS, Rosengart TK, Chatterjee S, Ghanta RK. Development of a Machine Learning Model to Predict Outcomes and Cost After Cardiac Surgery. Ann Thorac Surg. 2022 Jul 30;S0003-4975(22)00997-3. doi: 10.1016/j.athoracsur.2022.06.055.
News Story By Jeanie F. Woodruff, BS, ELS