Dr. Matthew Segar Recognized With DEI Abstract Award From Heart Rhythm Society
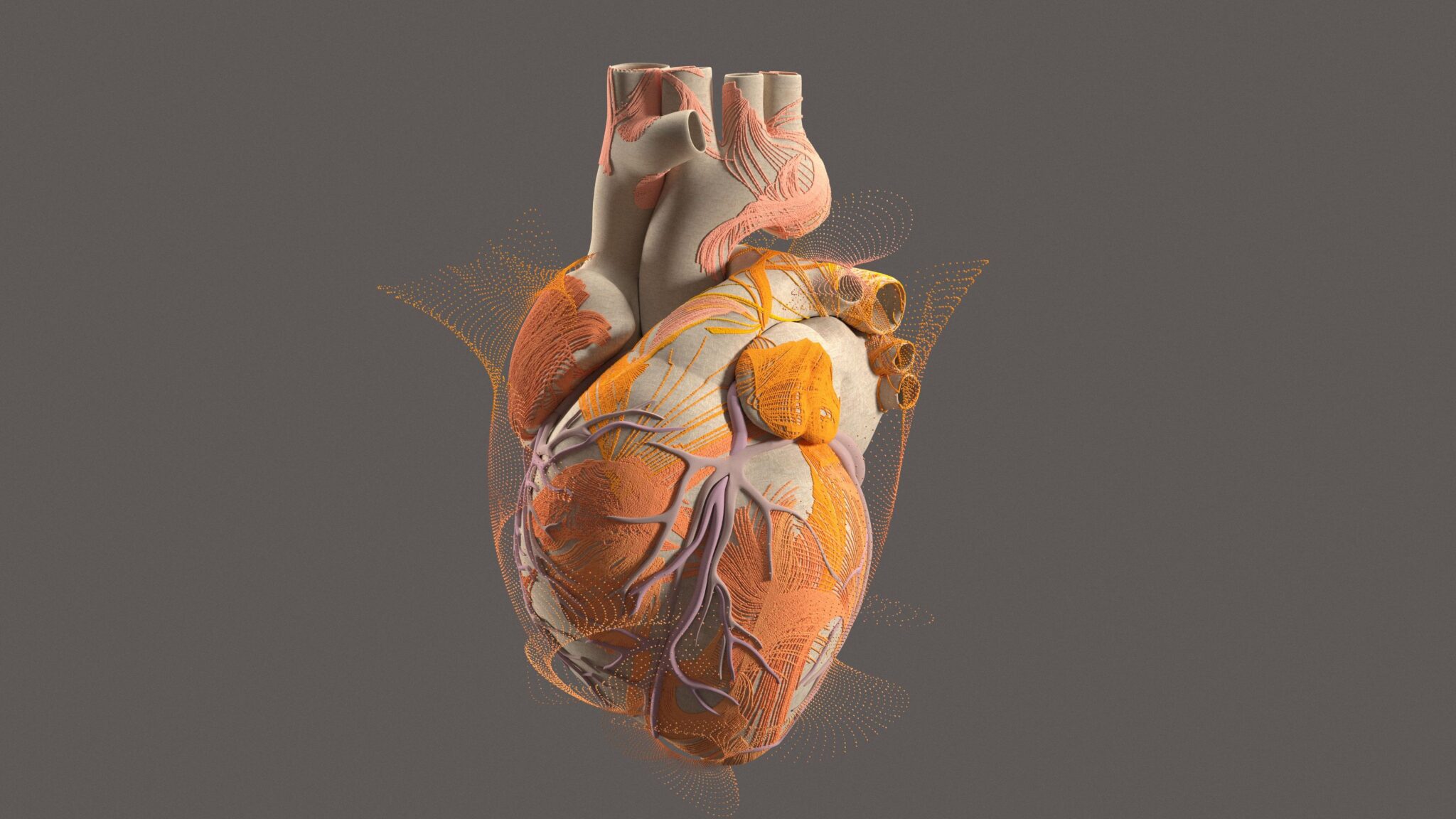
Novel Machine Learning Model Improves Prediction of Atrial Fibrillation Risk
The Heart Rhythm Society recently recognized Matthew Segar, MD, MS, for his contribution to a new machine learning-based model to predict atrial fibrillation. The model improved fairness in atrial fibrillation risk prediction across races and outperformed a traditional risk score for the condition.
The professional society selected the abstract summarizing the new model as one of five DEI Abstract Award Finalists. Dr. Segar took second place overall in the competition and won the oral presentation award for his talk on May 17 at the Heart Rhythm 2024 meeting in Boston, Massachusetts.
Dr. Segar is completing his third year as a fellow in The Texas Heart Institute Cardiovascular Disease Fellowship at Baylor St. Luke’s Medical Center. His abstract co-authors constitute a collaborative, multidisciplinary team of investigators from institutions across Texas and the United States, with collective expertise in cardiovascular disease and cardiac electrophysiology, clinical trials, preventive medicine, risk assessment, health disparities research and access to care, and biostatistics and data science. Cardiac electrophysiologists Drs. Mehdi Razavi and Mohammad Saeed of The Texas Heart Institute and Baylor College of Medicine are among the co-authors of the study.
Atrial fibrillation is a heart-rhythm disorder that affects approximately 6 million people in the U.S. and can increase a person’s risk of stroke, heart failure and sudden cardiac arrest. Current methods used to calculate atrial fibrillation risk include race as a factor and may contribute to racial disparities in treatment. To help reduce these disparities and improve the accuracy of risk prediction, the investigators developed a new machine learning computer model—one that uses artificial intelligence to find patterns within datasets—that is race agnostic (i.e., does not use race as a factor). Using large sets of patient data to develop the model, risk predictions were based on a person’s age, health factors, lab results, social determinants of health (insurance, education), and other factors.
When Dr. Segar and his co-authors compared their machine learning model to the commonly used CHARGE-AF risk score, they found the new model did a better job of predicting atrial fibrillation risk and improved fairness across races. According to Dr. Segar, “Our machine learning model makes atrial fibrillation risk assessment more accurate and equitable across races. This allows for more inclusive clinical trial enrollment and targeted prevention, ultimately improving outcomes for all patients at risk for this condition.”
Read the Abstract
A Race-Agnostic Machine Learning-based Model Improves Incident Atrial Fibrillation Prediction
Matthew Segar, MD, MS (The Texas Heart Institute)
Byron C. Jaeger, PhD (Wake Forest University School of Medicine)
Kershaw V. Patel, MD (Houston Methodist)
Shreya Rao, MD, MPH (The University of Texas Health Science Center at San Antonio)
Mehdi Razavi, MD (The Texas Heart Institute and Baylor College of Medicine)
Mohammad Saeed, MD (The Texas Heart Institute and Baylor College of Medicine)
Utibe R. Essien, MD, MPH (David Geffen School of Medicine at the University of California, Los Angeles, and the Greater Los Angeles VA Healthcare System)
Ambarish Pandey, MD, MS (The University of Texas Southwestern Medical Center)
Heart Rhythm, Volume 21, Issue 5, Supplement, May 2024, Page S6, CE-482889-002
https://doi.org/10.1016/j.hrthm.2024.03.244